In the rapidly evolving world of technology, the ability to harness the power of machine learning has become increasingly crucial for businesses and organizations of all sizes. However, the complexity and technical expertise required to develop and deploy effective machine learning models have often been a barrier to entry for many. This is where Amazon SageMaker Autopilot steps in, revolutionizing the way we approach machine learning in the cloud.
Introduction to Sagemaker Autopilot
SageMaker Autopilot is a fully-managed machine learning service provided by Amazon Web Services (AWS) that aims to simplify the process of building, training, and deploying machine learning models. By automating many of the time-consuming and resource-intensive tasks associated with traditional machine learning, SageMaker Autopilot empowers users to focus on solving their business problems rather than getting bogged down in the technical complexities.
Automated Model Selection and Tuning
One of the key features of SageMaker Autopilot is its ability to automatically select and tune the most appropriate machine learning algorithm for a given dataset and problem. Instead of manually testing and comparing various algorithms, SageMaker Autopilot leverages its advanced capabilities to explore a wide range of models, evaluate their performance, and identify the optimal solution.
No-Code/Low-Code Approach
SageMaker Autopilot’s user-friendly interface and intuitive design make it accessible to users with varying levels of technical expertise. Whether you’re an experienced data scientist or a business analyst with little coding experience, SageMaker Autopilot’s no-code/low-code approach allows you to harness the power of machine learning without the need for extensive programming knowledge.
Scalable and Efficient
As a cloud-based service, SageMaker Autopilot provides users with the scalability and efficiency needed to handle large-scale machine learning tasks. The service automatically provisions and manages the necessary computing resources, allowing users to focus on the problem at hand rather than worrying about the underlying infrastructure.
Benefits of using Sagemaker Autopilot
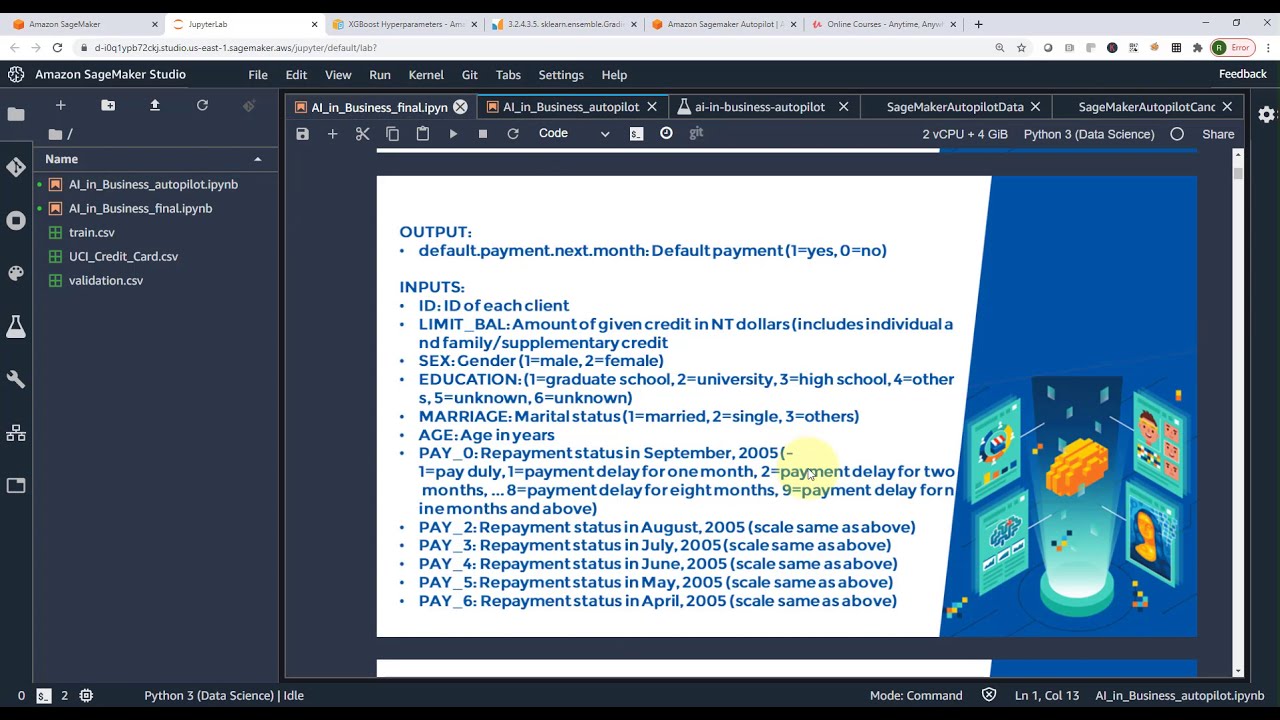
Adopting SageMaker Autopilot can bring a range of benefits to organizations, from improved efficiency and cost-effectiveness to accelerated model development and deployment.
Reduced Time-to-Value
By automating the model selection and tuning process, SageMaker Autopilot significantly reduces the time required to develop and deploy machine learning models. This translates to faster time-to-value for businesses, allowing them to quickly implement machine learning solutions and start realizing the benefits.
Increased Productivity
SageMaker Autopilot’s automated workflows and user-friendly interface free up valuable time and resources for data scientists and developers, enabling them to focus on more strategic and high-impact tasks. This increased productivity can lead to improved outcomes and a greater return on investment.
Reduced Technical Complexity
The technical complexities associated with traditional machine learning can be a significant barrier to entry, especially for organizations with limited data science expertise. SageMaker Autopilot’s no-code/low-code approach removes these barriers, making machine learning accessible to a wider range of users.
Improved Model Performance
SageMaker Autopilot’s automated model selection and tuning capabilities often result in better-performing models compared to those developed through manual processes. By exploring a wider range of algorithms and hyperparameters, the service can identify solutions that are more tailored to the specific problem at hand.
Scalability and Flexibility
As a cloud-based service, SageMaker Autopilot offers the scalability and flexibility needed to handle growing data volumes and evolving business requirements. Users can easily scale their machine learning workloads up or down as needed, without the need for complex infrastructure management.
How Sagemaker Autopilot works

SageMaker Autopilot’s workflow can be broadly divided into several key steps, each designed to streamline the machine learning development process.
Data Preparation
The first step in the SageMaker Autopilot workflow is data preparation. Users upload their dataset to an Amazon S3 bucket, and the service automatically analyzes the data, identifying any issues or missing values that need to be addressed.
Automatic Feature Engineering
Once the data is prepared, SageMaker Autopilot’s automated feature engineering capabilities come into play. The service analyzes the dataset, identifies relevant features, and generates new features that can improve the model’s performance.
Algorithm Selection and Tuning
Using its advanced machine learning algorithms and hyperparameter optimization techniques, SageMaker Autopilot then selects the most appropriate model for the task at hand. The service evaluates a wide range of algorithms, including popular choices like linear regression, random forest, and gradient boosting, to determine the optimal solution.
Model Training and Evaluation
With the selected algorithm, SageMaker Autopilot automatically trains the machine learning model and evaluates its performance using various metrics, such as accuracy, F1-score, and area under the ROC curve.
Deployment and Monitoring
Once the model has been trained and evaluated, SageMaker Autopilot facilitates the deployment of the model to a production environment, where it can be used to make predictions or inferences. The service also provides monitoring capabilities to ensure the model continues to perform well over time.
Continuous Improvement
SageMaker Autopilot’s iterative approach allows for continuous improvement of the machine learning model. As new data becomes available or the business requirements change, the service can be used to retrain and fine-tune the model, ensuring it remains effective and up-to-date.
Case studies/examples of successful use
SageMaker Autopilot has been successfully adopted by a wide range of organizations, from startups to large enterprises, across various industries. Here are a few examples of how SageMaker Autopilot has been used to drive real-world business outcomes.
Predicting Customer Churn for a Telecommunications Company
A leading telecommunications company used SageMaker Autopilot to develop a machine learning model for predicting customer churn. By automating the model selection and tuning process, the company was able to quickly identify the most effective algorithm and deploy the model in production, leading to a significant reduction in customer churn and increased customer retention.
Optimizing Inventory Management for an E-commerce Retailer
An e-commerce retailer leveraged SageMaker Autopilot to build a machine learning model for forecasting product demand and optimizing inventory levels. The automated feature engineering and model tuning capabilities of SageMaker Autopilot enabled the retailer to develop a highly accurate model that reduced inventory costs, improved product availability, and enhanced overall operational efficiency.
Automating Fraud Detection for a Financial Institution
A financial institution used SageMaker Autopilot to develop a machine learning-based fraud detection system. The service’s ability to quickly explore and evaluate a wide range of algorithms allowed the institution to implement a highly accurate fraud detection model, leading to a significant reduction in financial losses and improved customer trust.
Predicting Equipment Failures for a Manufacturing Company
A manufacturing company utilized SageMaker Autopilot to build a predictive maintenance model for their industrial equipment. By automating the model development process, the company was able to identify the optimal machine learning algorithms and deploy the model to proactively detect and address equipment failures, reducing downtime and maintenance costs.
Comparison to traditional machine learning methods
While traditional machine learning methods have their place, SageMaker Autopilot offers several distinct advantages that set it apart from more manual approaches.
Reduced Complexity and Technical Expertise
Traditional machine learning often requires a deep understanding of programming, statistics, and machine learning algorithms. SageMaker Autopilot’s no-code/low-code approach, on the other hand, makes machine learning accessible to a wider range of users, including those with limited technical expertise.
Faster Model Development and Deployment
Developing and tuning machine learning models through traditional methods can be a time-consuming and resource-intensive process. SageMaker Autopilot’s automated workflows significantly reduce the time required to go from data to deployed model, enabling organizations to realize the benefits of machine learning more quickly.
Increased Scalability and Efficiency
As a cloud-based service, SageMaker Autopilot provides users with the scalability and computing power needed to handle large-scale machine learning tasks. Traditional on-premises solutions may struggle to keep up with growing data volumes and computational demands.
Continuous Improvement and Adaptation
SageMaker Autopilot’s iterative approach to model development and deployment allows for continuous improvement and adaptation to changing business requirements. Traditional machine learning methods may require more manual effort to update and fine-tune models over time.
Cost-Effectiveness
By automating many of the time-consuming and resource-intensive tasks associated with machine learning, SageMaker Autopilot can ultimately lead to cost savings for organizations, both in terms of reduced labor and computing infrastructure costs.
Future potential and developments
As machine learning continues to revolutionize the way we approach problem-solving, the future potential of SageMaker Autopilot is truly exciting. Here are some of the key developments and advancements that we can expect to see in the years to come.
Expanded Algorithm and Model Support
As the field of machine learning rapidly evolves, we can expect SageMaker Autopilot to continuously expand its library of supported algorithms and model types. This will enable the service to handle an even wider range of use cases and problem domains.
Improved Explainability and Interpretability
One of the challenges with many machine learning models is their inherent “black box” nature, making it difficult to understand the reasoning behind their predictions. Future developments in SageMaker Autopilot may focus on improving the explainability and interpretability of the models it generates, providing users with greater transparency and insights.
Integration with Other AWS Services
As part of the broader AWS ecosystem, SageMaker Autopilot is well-positioned to integrate seamlessly with other AWS services, such as Amazon Athena for data exploration, Amazon Redshift for data warehousing, and Amazon Kinesis for real-time data processing. This integration will further streamline the machine learning workflow and enable more comprehensive, end-to-end solutions.
Increased Automation and Intelligence
SageMaker Autopilot’s current level of automation is already impressive, but we can expect to see even more advanced levels of intelligence and automation in the future. This may include capabilities like automatic dataset curation, intelligent feature engineering, and self-improving model performance.
Enhanced Edge and IoT Deployments
As the demand for machine learning at the edge and in IoT (Internet of Things) devices continues to grow, SageMaker Autopilot may evolve to support more seamless deployments in these environments. This could involve optimizing model size and performance for deployment on resource-constrained devices.
Conclusion
In the rapidly evolving world of machine learning, Amazon SageMaker Autopilot stands out as a game-changing service that revolutionizes the way organizations approach and implement data-driven solutions. By automating the complex and time-consuming tasks associated with traditional machine learning, SageMaker Autopilot empowers users of all skill levels to harness the power of artificial intelligence and accelerate their path to real-world business impact.
Through its automated model selection, feature engineering, and deployment capabilities, SageMaker Autopilot has proven to be a valuable tool for organizations across a wide range of industries, from telecommunications and e-commerce to financial services and manufacturing. As the field of machine learning continues to evolve, the future potential of SageMaker Autopilot is truly exciting, with the promise of even greater automation, intelligence, and integration with the broader AWS ecosystem.
For organizations looking to unlock the transformative power of machine learning, SageMaker Autopilot offers a compelling solution that simplifies the process, reduces technical complexity, and enables faster time-to-value. By leveraging this innovative service, businesses can focus on solving their most pressing challenges, secure in the knowledge that the underlying machine learning infrastructure is being handled seamlessly in the cloud.